How voice recognition will change the world
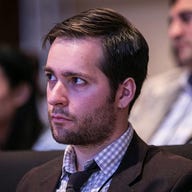
It's Friday morning and you're thinking about rewarding a week's worth of hard work by escaping the fluorescent lights of the office for a lunch hour in the sun.
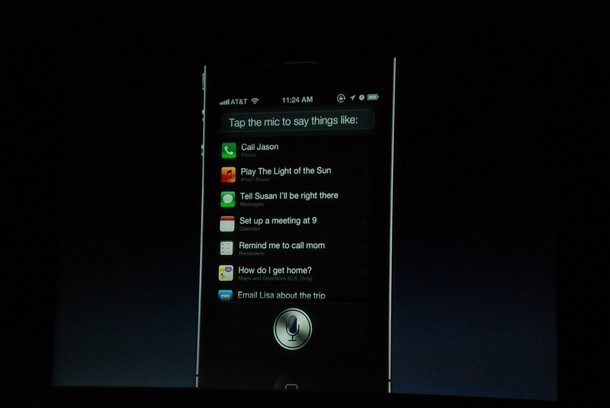
Siri
(Credit: CNET)
Eating alone is no fun, of course, so you might as well invite a good friend.
You pick up your new Apple iPhone 4S smartphone and press and hold the home button.
At the bottom of the screen, a little microphone appears. "What can I help you with?" it asks.
It beeps. You begin to speak.
"Send text to Erica: Let's have lunch."
In an instant, your intended message appears as tech on the screen, loaded into an abbreviated version of the phone's standard SMS form. Erica's name has populated the necessary field. Without missing a beat, it asks: "Ready to send it?" You reply "Yes". And off it goes.
It couldn't be easier, short of the phone reading your mind. The entire process takes three seconds at most.
In reality, the process you just experienced is the culmination of decades of intense interdisciplinary research. What was a nearly seamless transaction between your mouth and your phone was, in fact, a complex series of computational decisions designed to understand what took you, as an infant, years to master: language.
In the last 70 years, computers have amazed us with their ability to conduct simple calculations at astonishing speeds. With each passing decade, the computer's ability to process these calculations has advanced to the point where today, we are tasking it with our most pressing, complex problems: mapping global climate change, particle physics, materials science and more at the atomic level and beyond. Things that would take hundreds of humans hundreds of lifetimes to calculate on their own.
All of these phenomena operate under a strict series of laws, of course. Our ability to understand them is only as sufficient as our ability to assemble a large enough data set to represent them. And aside from the occasional once-in-a-lifetime discovery that redefines those rules — such as when scientists discovered bacteria last year that survived on arsenic, an element not among the six known to constitute life — the rules remain constant.
But language, that's a different story. Language is, essentially, a complex system of communication — but it's developed by humans, not the natural world, and certainly not by a computer operating in a binary existence. It is, then, inherently imperfect; it develops in different ways at different rates and it does not subscribe to a firm set of rules. In the world of computers and Mother Nature, slang like "You be illin'" is an anomaly of epic proportions. Multiple words for a single meaning collide with multiple meanings for a single word. Speaking it aloud complicates understanding even further. And worst of all, the rules (and accents) for the more than 3000 languages spoken worldwide are in a constant state of change.
It is, therefore, a tremendous feat to marry the rigid world of computers to the squishy, volatile world of spoken language. And yet your new smartphone just managed to accomplish it in seconds.
From breath to bytes
So what really happened after you pressed that button? It goes something like this:
The sounds of your speech were immediately encoded into a compact digital form that preserves its information.
The signal from your connected phone was relayed wirelessly through a nearby cell tower and through a series of land lines back to your internet service provider where it then communicated with a server in the cloud, loaded with a series of models honed to comprehend language.
Simultaneously, your speech was evaluated locally, on your device. A recogniser installed on your phone communicates with that server in the cloud to gauge whether the command can be best handled locally — such as if you had asked it to play a song on your phone — or if it must connect to the network for further assistance. (If the local recogniser deems its model sufficient to process your speech, it tells the server in the cloud that it is no longer needed: "Thanks very much, we're OK here.")
The server compares your speech against a statistical model to estimate, based on the sounds you spoke and the order in which you spoke them, what letters might constitute it. (At the same time, the local recogniser compares your speech to an abridged version of that statistical model.) For both, the highest-probability estimates get the go-ahead.
Based on these opinions, your speech — now understood as a series of vowels and consonants — is then run through a language model, which estimates the words that your speech is comprised of. Given a sufficient level of confidence, the computer then creates a candidate list of interpretations for what the sequence of words in your speech might mean.
If there is enough confidence in this result, and there is — the computer determines that your intent is to send an SMS, Erica Olssen is your addressee (and therefore her contact information should be pulled from your phone's contact list) and the rest is your actual note to her — your text message magically appears on screen, no hands necessary. If your speech is too ambiguous at any point during the process, the computers will defer to you, the user: did you mean Erica Olssen, or Erica Schmidt?
All this, in the space of three seconds.
Talk to me
Developing a machine that understands the spoken word has intrigued engineers and scientists for centuries.
It was certainly on the mind of Bell Laboratories research physicist Homer Dudley in 1940, when he was granted a patent for the "Parallel Bandpass Vocoder", a device that analysed the energy levels of sound samples through a series of narrow band filters, then reversed the process by scanning the results and sending them to more filters that were attached to a noise generator.
The year prior, Dudley received a patent for his Voder speech synthesiser, a valve-driven machine that modelled the human voice with the help of a human operator manipulating various controls. It built on years of research conducted by his colleague Harvey Fletcher, a physicist whose work in the transmission and reproduction of sound firmly established the relationship between the energy of speech within a frequency spectrum and the result as perceived by a human listener. (Most modern algorithms for speech recognition are still based on this concept.)
But it wasn't until 1952 that Bell Laboratories researchers developed a system to actually recognise, rather than reproduce or imitate, speech. K.H. Davis, R. Biddulph and S. Balashek devised a system to recognise isolated digits spoken by a single person. Much like its digital successors, the system estimated utterances (eg, the word "nine") by measuring their frequencies and comparing them to a reference pattern for each digit to guess the most appropriate answer.
"Historically, there is evidence that mankind has been very interested in automation of interfacing with the world around us," said David Nahamoo, IBM fellow and the company's chief technical officer for speech. "Some people point to things a hundred years old that could touch on speech technologies today."
Advancements quickly followed. In 1956, Harry Olson and Herbert Belar of RCA Laboratories developed a machine that recognised 10 syllables of a single talker. In 1959, James and Carma Forgie of MIT Lincoln Lab developed a 10-vowel system that was speaker-independent; the same year, University College researchers Dennis Fry and Peter Denes focused on developing a recogniser for words consisting of two or more phonemes — the first use of statistical syntax at the phoneme level in speech recognition.
Development of analog systems based on spectral resonances accelerated in the 1960s. In 1961, IBM researchers developed the "Shoebox", a device that recognised single digits and 16 spoken words. In 1962, Jouji Suzuki and Kazuo Nakata of the Radio Research Lab in Tokyo, Japan, built a hardware vowel recogniser while Toshiyuki Sakai and Shuji Doshita at Kyoto University built a hardware phoneme recogniser — the first use of a speech segmented for analysis. The following year, NEC Laboratories developed a hardware digit recogniser. And Thomas Martin of RCA Laboratories developed several solutions to detect the beginnings and endpoints of speech, boosting accuracy.
"The limitation was essentially man in the loop," Nahamoo said. "To build anything, a person had to sit down, look at a visual representation of a signal that was spoken for a given word, and find some characteristics — a signature — to then write a program to recognise them. Based on that, a reverse process could be done. This was extremely slow because the discovery of those characteristics by a human brain was taking a long time. That was the main roadblock. The characteristics — the specifics that would give away a sound or word — had to be discovered automatically. Human discovery was very slow."
Encoding conversation
By the late 1960s and 1970s, modern computers had emerged as a way to automatically process signals, and a number of major research organisations tasked themselves with furthering speech recognition technology, including IBM, Bell, NEC, the US Department of Defense and Carnegie Mellon University. Scientists developed various techniques to improve recognition, including template-based isolated word recognition, dynamic time warping and continuous speech recognition through dynamic phoneme tracking.
"A lot of the interest in speech recognition was driven by DARPA," Nahamoo said of the US Department of Defense's research arm. "DARPA was actively funding it from the early days, and its sister organisations in government naturally had their own reasons for their interest. The difference between government and industry was that the industry from a business perspective was not under pressure to replace the GUI — graphical user interface. But government had the need to be able to process massive amounts of spoken content in different form and fashion to be able to extract insight from it. They drove the industrial institution as well as universities to help scientists innovate."
The period also marked the first use of sophisticated computer algorithms, which helped better accommodate multiple speakers, include semantic information and reference a growing database of vocabulary.
"It was very clear that to move the things from science to engineering, we needed the computers," Nahamoo said. "For scientific work, the human brain was actually designed better than our computers. But for many engineering things where you had to search and space words and do modelling, they helped. When I joined IBM, what was state-of-the-art is weaker than the iPhone you use today."
The 1980s brought a shift in methodology from basic pattern recognition to statistical modelling in an attempt to recognise a fluently spoken string of connected words. Each organisation had its own reasons to further research in the field: the US Department of Defense sought to lead research and development in the lab; IBM sought to produce a voice-activated typewriter for office transcription; AT&T Bell sought to provide automated telecommunication services such as voice dialling and phone call routing. In all cases, speaker-independent systems were preferred and mathematical rigour was the method of choice.
"The computers made things systematic," Nahamoo said. "You could repeat it and plot it and tabulate, organise and model data. It allows us to build more and more refined models of different things. We have three orders of magnitude more complexity today than we did in the early 1970s. They gave us the ability to refine the model."
From research lab to real life
The 1990s brought a sharp focus on pattern recognition and a proliferation of use cases, from air travel information requests to broadcast news transcriptions to recognising spontaneous, conversational speech. It was here that the most difficult challenges, "disfluencies", were confronted: speakers who cut themselves off mid-word, hesitate or correct themselves, not to mention handling the various non-word fillers that pepper casual speech, such as "uh" and "um".
As humans and machines stepped closer to each other, a renewed focus on two-way conversation — including the management of ambient noise — emerged. Researchers believed that the speech-to-text process was the optimal first step to allow recognition systems to respond; Massachusetts Institute of Technology scientists developed the Pegasus and Jupiter systems as a result, which offered airline information and weather information, respectively. AT&T's How May I Help You system and later, its Voice Recognition Call Processing system, successfully cut down on the escalating expense of call centres by allowing machines to handle basic requests.
"In human history, speech has essentially played three roles: it has been useful for communicating and interacting; it has been useful for doing monologues, that is, creating content that can be understood; and third, it has been useful as a barometer to give away the identity or verification of a person," Nahamoo said. "From a speech recognition standpoint, we are now starting to expect a dialogue with an automated system. While early dialogues were based on directed dialogues of IVR, we are now moving into the next generation where dialogues are expected to be a lot more free-form. That's what you're starting to experience in consumer applications like Siri and Dragon Go."
With every passing year, speech recognition moved further from its beginnings as a novelty and closer to its business role as a time- and money-saving tool.
"In the scientific world, we have dealt with these things for the last 15 years, but they have all been in the exploration phase," Nahamoo said. "In the next couple of years, because of mobility, and because of smart devices, the necessity for this technology has become very clear and obvious. We're just at the start of this journey."
Microsoft's mission
It's a miserable December day in Manhattan and pedestrians up and down Lexington Avenue are ducking under scaffolding and scampering down the footpath in an attempt to shield themselves from the rain.
I'm standing inside the sleek lobby of the W Hotel, looking somewhat like the drowned rat I spotted in the subway on my way uptown. I'm waiting to meet with Zig Serafin, general manager of the Speech at Microsoft group. When I make it to the right room, I knock on the door and enter. Zig's not here — he's sitting in his office in surprisingly sunny Redmond, Washington. But with a little help from an Xbox, we're quickly in a video-conference — and from the way he's looking at me, he's probably wondering why anyone would want to live in precipitation-heavy New York City.
But no matter; we're here to talk about that Xbox. What began as a console gaming platform has become the company's pipeline into the living room, and what used to be a way to waste some time on the weekend has now, with the help of the sensor-laden Kinect accessory, become a proving ground for some of Microsoft's latest advancements on the natural user interface.
You see, the Kinect can hear you (as we're demonstrating through our video chat), see you (ditto) and understand you — it follows basic spoken commands from across the room.
"There are places where your voice is most appropriate to use, like the living room," he said. "There are places where it's more appropriate to use a gesture. It's not just an interface island. You want an experience based upon the modality that's most appropriate to the device or screen you're dealing with."
As we continue talking about how humans interact with technology, I begin to piece things together. A month before, Microsoft launched its Windows Phone mobile platform, catapulting the company back into the smartphone race. It remains a dominant player in the PC sector. Its free BING-411 service — a directory assistance line named after its search engine and launched in 2007 — was powered by Tellme, the former name of Serafin's group. And it has quickly become a major player in the automotive industry by partnering with Ford and Kia to offer a voice-activated telematics system that turns the phrase "I'm hungry" into an action item.
All of these products have voice recognition services available for users. All, I realise, are nodes through which Microsoft can collect data to improve its system. Empowered by the proliferation of internet connectivity, Microsoft is circling the wagons on voice recognition. And few consumers are aware it's even happening.
"We don't have a bunch of separate feedback loops; we have a whole bunch of fishing lines out there to bring back fish to one place," said Larry Heck, chief scientist of Microsoft's speech group. "People are using speech recognition in the car, while walking down the street with a smartphone in their hand, when they're in a cafe."
Speech recognition research is hardly new at Microsoft. The company pursued it under Bill Gates' leadership beginning in the early 1990s and through a series of acquisitions — Entropic in 1999 for server-based speech recognition, TellMe for voice services in 2007 — slowly commercialised what had long been an R&D pursuit.
Aside from the implementation of the technology in its most popular consumer devices, the company also runs between 65 and 70 per cent of all directory assistance requests in the US. Various voices, accents, ambient noise conditions and, of course, requests — it is an enormous and constantly-growing source of data.
"You could ultimately build a smarter system, one that improves itself faster than what's in the marketplace," Serafin said. "You're effectively sharpening the brain of the underlying system. That's when we put on turbo thrust to understand the conversational understanding speech system. What happens when you bring the ear together with the brain to hear and process what someone says accurately, then vocalise back with the mouth? All of this comes from the combination of speech recognition, speech synthesis, language understanding and text-to-speech analysis under one roof."
Google's goal
Across town, Google is also pursuing voice recognition through the development of its own system. During a visit to the company's office in New York's Chelsea neighbourhood, speech technology chief Mike Cohen told me that the company has been working in the area for seven years, primarily because of its utility in the mobile space — "the killer app for speech technology", as he describes it.
"Google's mission is to organise the world's information and make it easily accessible," he said. "Turns out [that] a lot of the world's information is spoken."
Google, of course, is not merely the company behind its namesake search engine for the web, but also behind the Android mobile operating system, which appears on smartphones, tablet computers and its Google TV product. Like Microsoft, it too had a free 411 service, GOOG-411, that it launched in 2007. (It discontinued the service in late 2010.) And while it hasn't made any formal announcements in the automotive space, its mobile OS has been used in a number of aftermarket infotainment products for cars.
"A big long-term mission for us is being ubiquitous," Cohen said.
Cohen tells me the 411 service was the company's first significant source of data, and that mobile speech input has grown 600 per cent in the last year. With each passing day, the company collects enough speech data to fill the space of two years.
The company's tentacles for voice recognition are spread across five categories: voice search (desktop, mobile and in its Chrome web browser), voice actions (for local content, through GPS and SMS), voice input (through the microphone button on Google Android phones), speech-to-speech translation (mobile) and spoken information (voicemail, YouTube video transcription).
The most growth by far is in the voice input category, which required "a lot of work under the hood" to offer, Cohen said. There is some data sharing between the categories, but some like YouTube are still somewhat siloed from the rest because the data is so different.
As Google builds its speech database and refines its models, Cohen admitted that it's learning a lot. For example, there are many variables: environmental conditions vary, there can be different microphones on different devices and there are even gender differences — women have a shorter vocal tract, making comprehension of that speech data slightly different from data of male speech.
The big goal: transparent computing, where the mode of interaction is the one that's most natural and in which the layers of technology between user and solution are reduced as much as possible. Or as Cohen describes it, "frictionless performance".
"The principle here is, the user is using his or her speech to shorten the distance between their intent and their desired outcome," said Vlad Sejnoha, chief technology officer at Nuance Communications. "In a lot of cases, we can accomplish that readily in one utterance. More and more we're applying natural language speech understanding as a real meaningful complement to visual interfaces."
The nuance factor
Sejnoha's company, of course, is the elephant in the speech recognition room. With US$1.4 billion in revenue — 90 per cent from voice services — the company is dominant in the space, supported by three pillars: transcription, call centre support and mobile environments.
While the company makes the most money in the first category — Tenet and Kaiser are among its clients — it's the third that's the most interesting. The company's technology is in everything from the T-Mobile MyTouch 4G to the in-car telematics systems offered by Ford, GM, Toyota, Honda, Audi, Mercedes and Volvo. It supplies technology to mobile products made by Apple, Nokia, Motorola, Samsung, HTC and LG; it's in GPS navigation devices from Garmin, TomTom and Magellan; and its technology is even embedded in set-top boxes from American Scientific and Motorola.
The company has benefited greatly from a tight relationship with speech pioneer IBM, whose extensive patent portfolio Nuance licenses for commercial use. (The company purchased a portion of that portfolio in 2009.) Now it's finding new places to implement voice-based interaction.
"A user might connect to our system through a number of points: your phone, your tablet, your car, your desk, when you contact enterprise care," Sejnoha said. "Not only do we want to aggregate those learnings and improve your experience everywhere — remove those silos — but also provide a coherent experience across those endpoints."
A profitable platform
In the age of the internet, the speech recognition race has quickened — and the winners are those who assemble the most data.
"With these things — Watson, Siri, Dragon Go — people are starting to see that [some interactions are] going to happen in some linguistic fashion," Nahamoo said. "The question is, what aspects of these technologies is going to become a keyboard — a commodity where it's unclear where the business is — versus applications that exist that are the underpinning of business?"
The stakes are high. As globalisation becomes the economic norm, the capability of technology to manage and manipulate language is paramount. It's not just in consumer electronics: large call centre operations continue to employ speech recognition to process requests and time-crunched doctors around the world rely on it to dictate notes over the phone for transcription.
"The big theme here is we are entering an era where more and more people are understanding that speech [recognition] is no longer this add-on that makes text," Sejnoha said. "Natural language speech allows you to reach out and grab stuff."
The Internet of Things has always promised a fully connected world, but only hinted at what could be accomplished with it. In the future, you could be asking an automated teller machine for a balance, telling your smart home to lower the temperature by a couple of degrees or instructing your kitchen's coffee machine to make six cups on the strong side, milk and sugar.
Even better, your next call to customer service may actually detect how peeved you are — and respond accordingly.
"If things aren't going well, it's very useful to try to detect the emotional tone and interrupt an unsuccessful interaction," Sejnoha said, adding that people often call these systems "intelligent" even though they only perform computation, not deep reasoning like IBM's Watson supercomputer. "There's progress being made. There's useful stuff being done here."
Above all, the most impressive aspect of modern speech recognition is that system will constantly and automatically learn to improve itself. As smartphones proliferate around the globe, connected TV boxes make their way into the living room and intelligent cars hit the road, companies like Microsoft, Google and Nuance will see their datasets grow and grow with new sources of speech, from a Midwestern mum shouting over her children at the mall to a Bangladeshi cab driver sending text messages to friends as he speeds around New York City.
"Interest among Google, Microsoft and others is about how can they improve their search services," Nahamoo said. "I think these technologies to a good extent are enabling technologies, but they are not necessarily businesses unto themselves except for a small number of players. But their impact and implication in the world we live is going to be as important or more important as the telephone you use today. How business will arrive around that, well, be assured that a lot of creative people are working on this."
There's money to be made. Who will get to the endpoints first?
"The era of continuous improvement is here now," Sejnoha said. "It's just a matter of percolating through the market."
Via SmartPlanet